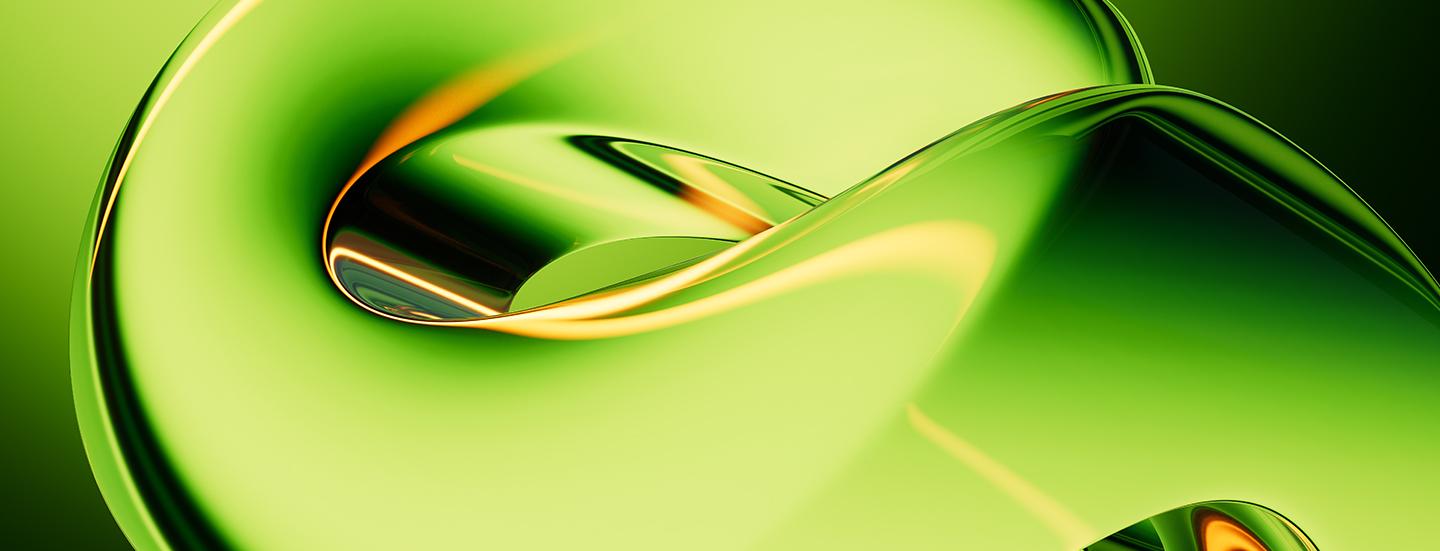
Enhancing MLR Review Efficiency in Pharma Through AI and Automation
Authored by Alfred Whitehead, MS, MAs
Introduction
The medical, legal, and regulatory (MLR) review process is central to maintaining compliance, accuracy, and ethical standards in pharmaceutical marketing and communications. Yet, lengthy reviews, frequent rework cycles, and large volumes of unused assets remain common pain points. These inefficiencies become even more pronounced when multiplied across global markets—each with unique regulations, languages, and local marketing strategies. Most companies hope to improve their content velocity, but MLR is far too complex to be solved with software alone.
Key Metrics and Challenges
A baseline understanding of performance is critical before optimizing MLR processes. Several core metrics can pinpoint areas ripe for improvement:
Throughput – How many assets clear review in a given time frame?
Review Time – How long does each stage of review take?
Time in Queue – How long do assets wait before active review?
Rework Cycles – How many revision rounds are needed before approval?
Post-Review Submission – How quickly do approved assets reach final submission (e.g., Form 2253)?
Beyond immediate efficiency gains, companies should also examine the hidden costs of unused assets. A systematic portfolio-management strategy can inform better decisions on creation and reuse, ensuring minimal waste and maximum value from existing materials.
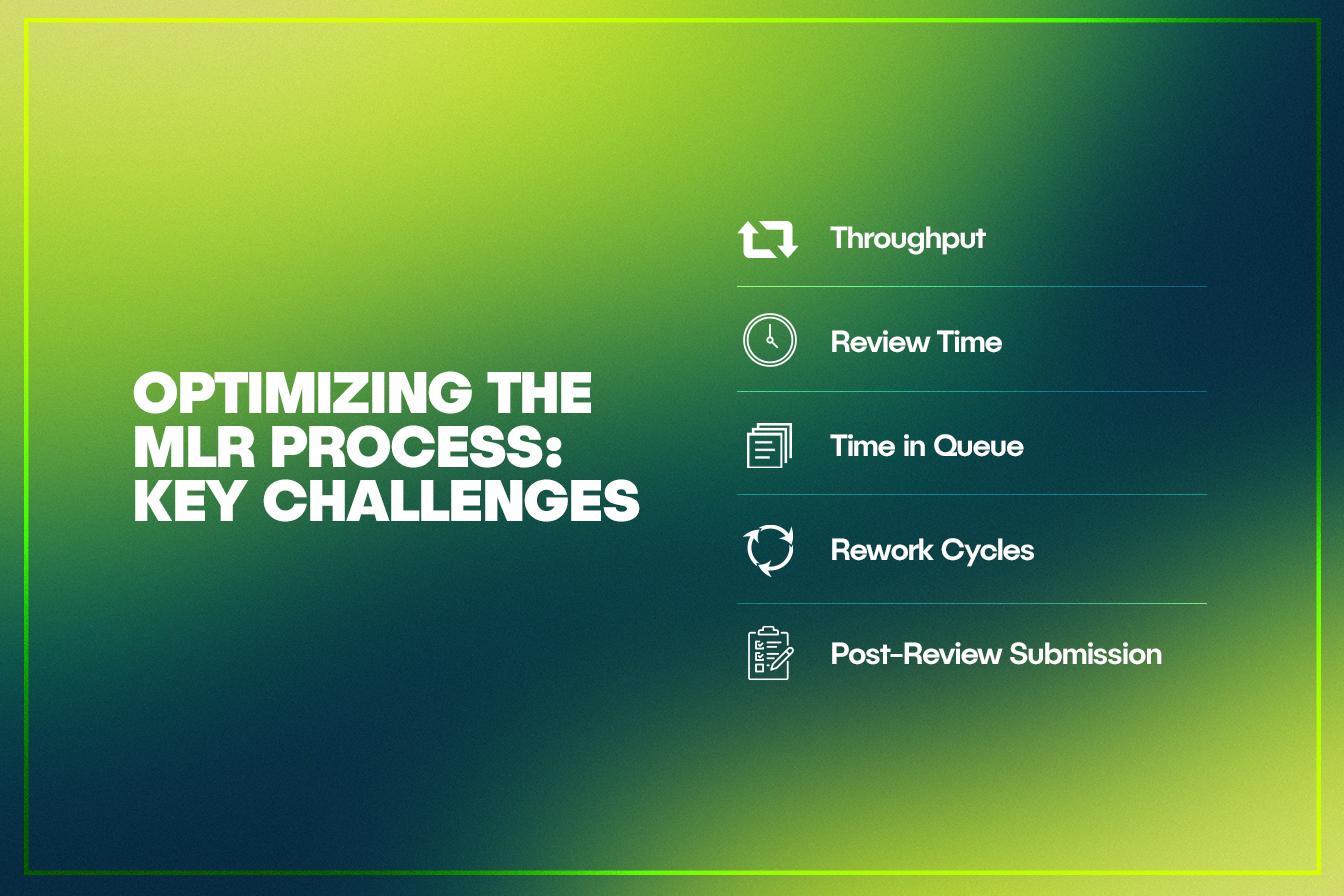
The Role of AI and Automation
AI and automation can alleviate many points of friction:
Real-Time Compliance Checks
Natural language processing (NLP) systems automatically flag risky language, fact-check claims against approved product labels and references, and streamline the review of frequently updated information.
Automated Workflows and Notifications
Smart routing and digital platforms reduce manual handoffs and administrative overhead. Teams receive triggers for review tasks, expiration notifications for previous approvals, and updates on shifting global regulations.
Early Integration in Content Creation
Embedding MLR requirements into AI-assisted authoring tools ensures materials begin in a compliant state, reducing revisions later on.
Leveraging AI for Global Content Reuse
For large international companies, content reuse is a significant opportunity to drive efficiency: Localized Variations
AI-based translation and localization tools can adapt preapproved content for new markets while preserving core messaging. Review times are shortened, and rework is reduced because content reuses existing regulatory and legal validations.
Regulatory Cross-Referencing
AI systems can map the differences between local regulations (e.g., FDA, EMA, TGA) and automatically highlight which elements of global content need adaptation. This activity speeds up time-to-market for global product launches.
Global Asset Libraries
Centralized repositories store previously approved content. AI-driven search and recommendation engines help teams quickly find and adapt assets that fit regional needs.
By making content reuse efficient and compliant, companies can scale their marketing efforts across diverse geographies without unnecessary duplication.
Implementation Barriers and Considerations
Incorporating AI and automation into the MLR review process requires overcoming several challenges. By setting clear KPIs, standardizing workflows, and ensuring high-quality data governance, pharmaceutical companies can build trust, align stakeholders, and unlock the full potential of AI-driven efficiencies.
Misaligned Incentives MLR teams are focused on mitigating risk, while commercial teams seek speed. Clear, shared KPIs (e.g., a target time-to-market that balances compliance and velocity) can harmonize these objectives.
Fragmented Teams and Workflows Coordinating MLR stakeholders across multiple regions and vendors can create communication breakdowns. Standardized, globally accessible platforms help align these groups.
Risk Aversion and AI Skepticism Concern over errors or biases introduced by AI can stall adoption. Rigorous pilot programs and phased rollouts can demonstrate reliability and build trust.
Pharma’s Reluctance to Be First Demonstrating clear success stories—especially within the same therapeutic area or regional market—can encourage broader adoption.
Data Quality and Governance AI is only as dependable as the data it ingests. Establish robust data governance, ensuring consistent, high-quality source information across global affiliates.
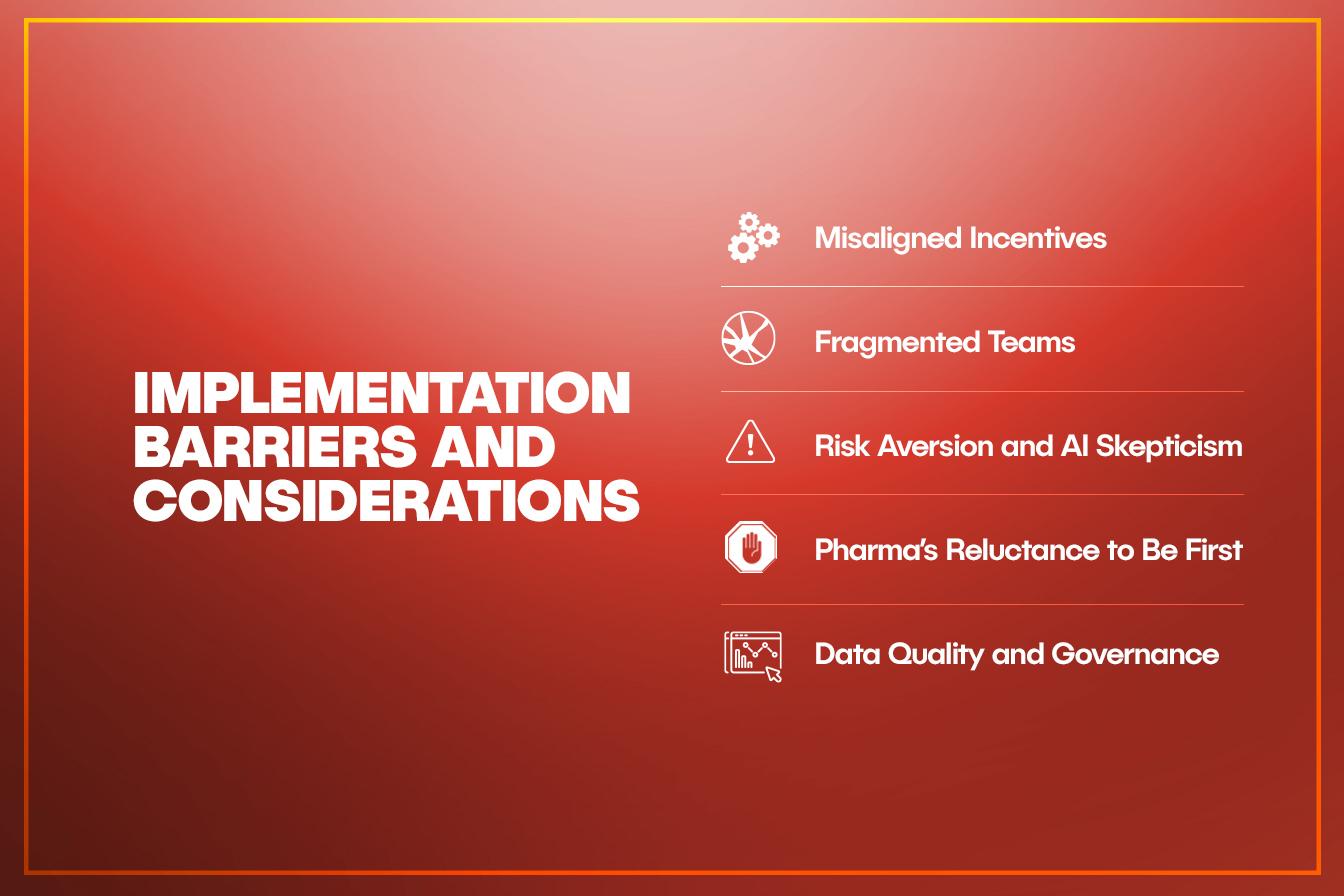
A Multilevel Maturity Model for MLR Optimization
Organizations can assess where they currently stand and chart a path toward advanced integration of AI and automation in MLR processes:
Level 1: Manual and Reactive
Little to no automation; heavy reliance on email and spreadsheets
Reviews are sequential and often siloed
Metrics are ad hoc or nonexistent
Level 2: Basic Automation and Metrics
Introduction of digital workflow tools to track reviews
Basic metrics capture (i.e., review times, number of cycles)
Early AI pilots (e.g., text matching for compliance checks)
Level 3: Integrated AI and Advanced Analytics
AI-driven tools for real-time compliance and content tagging
Consistent global asset library for reuse, powered by AI search
Cross-functional coordination with unified dashboards and KPIs
Multi-market alignment with localized content variations
Level 4: Predictive Compliance and Dynamic Global Orchestration
Predictive models flag high-risk content before creation begins
AI-driven adaptation of content for regional markets
Automated reporting and transparency, with minimal manual intervention
Continuous monitoring of performance metrics for proactive refinement
These levels often require gradual investment, culture change, and technology adoption. However, each level unlocks measurable gains in efficiency and compliance.
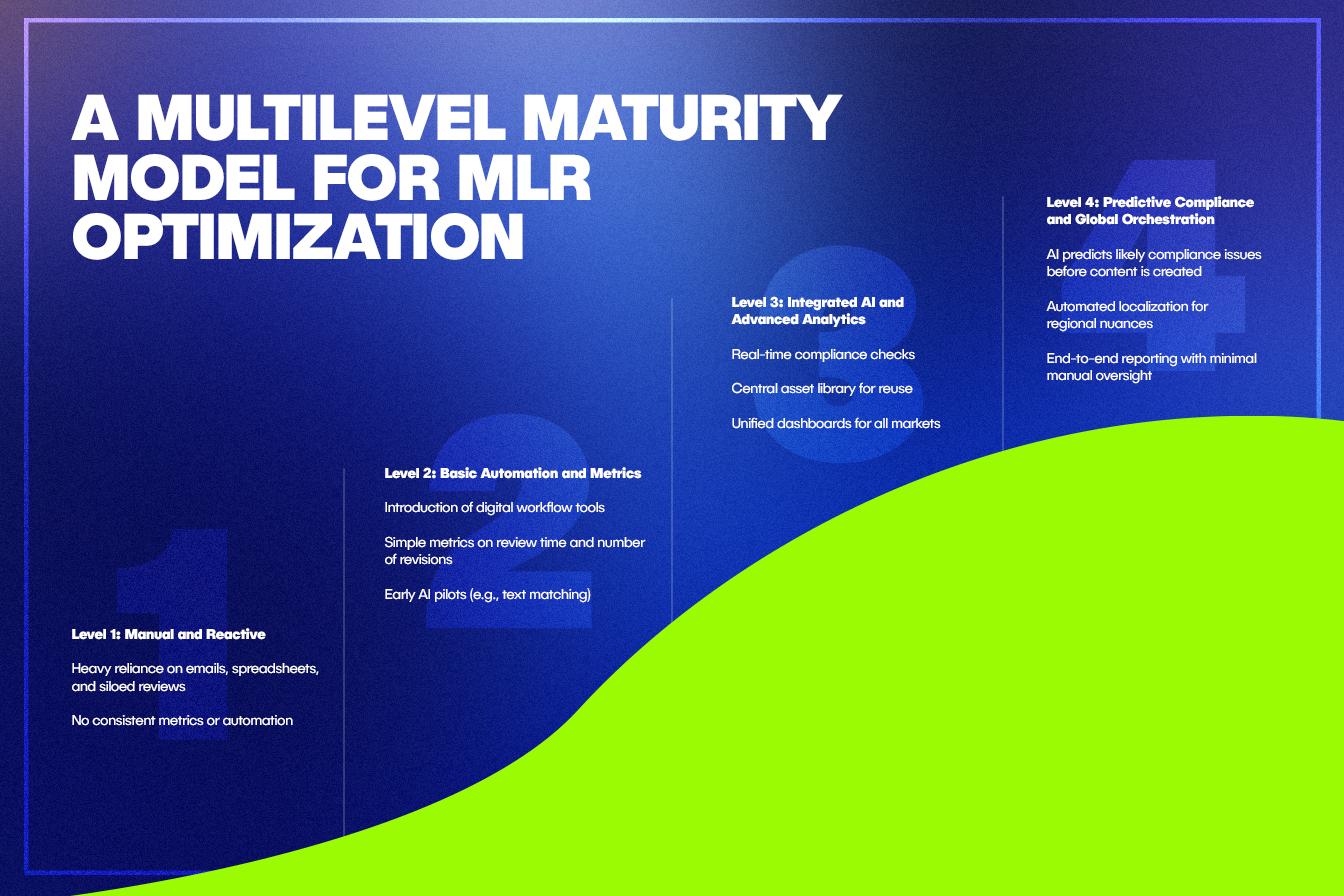
Emphasizing Measurement
Reliable metrics enable data-driven decisions. Before scaling AI, companies must implement consistent measurements that track:
Time saved per review cycle
Accuracy of AI-driven checks vs. human-only reviews
Frequency of content reuse across global markets
Commercial impact of extra days in market
These insights help teams identify areas for improvement and build a business case for deeper AI integration.
The Necessity of Human Oversight
As processes evolve, human expertise remains indispensable. While AI can manage large volumes of content, recommend edits, and track regulatory requirements, ultimate accountability and nuanced judgment cannot be automated. Ensuring an effective “human-in-the-loop” framework is essential for maintaining credibility and managing risk.
Future Outlook
Over the next five to 10 years, AI and automation will play an even larger role in MLR review:
Predictive Approvals AI will anticipate where non-compliance is likely to arise, prompting early intervention.
Multi-Market Synergy Local affiliates worldwide will seamlessly repurpose approved master content, minimizing duplication, while tailored compliance checks keep local nuances in view.
Portfolio-Level Optimization Advanced analytics will track asset usage, performance, and effectiveness, guiding smarter decisions about creation, retirement, and reuse.
The outcome is a more agile, globally consistent, and compliance-forward framework. By embracing robust measurement, collaborative culture, and strategic technology adoption, pharmaceutical companies can drive meaningful improvements in both speed-to-market and risk mitigation.
Conclusion
AI and automation present compelling opportunities for enhancing MLR reviews, particularly for global organizations looking to manage and reuse content efficiently across varied markets. Companies can follow a maturity model to implement these changes in a structured, risk-conscious manner. With the right balance of technology-driven automation and human expertise, MLR can become a competitive advantage—enabling faster delivery of critical information to healthcare providers and patients worldwide.
Author

Alfred Whitehead, MS, MAs
EVP, Applied Sciences
Alfred is a senior leader with over 15 years of experience in using data within the healthcare and life sciences industries. His team brings together behavioral science, medical science, data science, and both hardware and software prototyping to create novel solutions to problems affecting the improvement of human health. Alfred holds master's degrees in physics and astronomy, and is a published contributor to high-performance computer simulations in astrophysics.
Ready to Drive Life Sciences Forward?
Experience the transformative power of Klick Health, where deep industry expertise meets cutting-edge AI-driven wisdom.
As your trusted partners in life sciences commercialization, we combine a storied history in healthcare with the latest technologies to elevate every facet of your omnichannel strategy. From crafting engaging narratives to enabling data-driven decision-making, our integrated capabilities ensure you lead the way in transforming patient outcomes through digital health innovation.
Let’s create something transformative together.