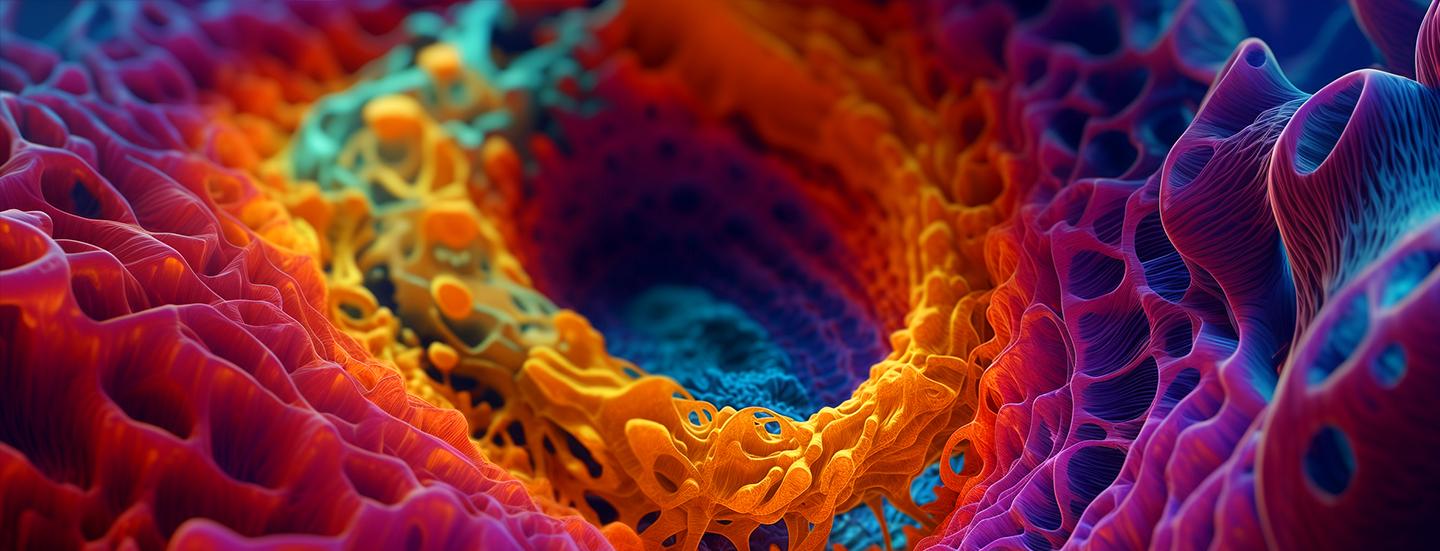
Hey everyone, this article is the first in a series that we’re calling “Generative AI: A Guide for Life Sciences Professionals.” As the name suggests, each week we’ll be breaking down a different aspect associated with GenAI and how it impacts all of us working in healthcare and the life sciences. First up, the ins and outs of unlocking business value using technology. Let’s dive in.
Navigating the vast array of generative AI tools and technologies can be daunting, making it difficult to determine the best starting point. You may be asking if it’s possible for your organization to test the waters with off-the-shelf solutions and still derive value, or do you need to go all in and create a custom model tailored to your specific needs? For executives grappling with these questions, this article aims to clear the fog.
We’ll explore these four common approaches to harnessing generative AI’s considerable power:
Utilize tools based on Large Language Models (LLMs)
Go deeper with Retrieval Augmented Generation (RAG)
Fine-tune a pre-existing model
Build a model from the ground up
The Versatile World of Large Language Models
Imagine a tool that can draft emails, write articles, or even answer customer queries in real time. Tools like ChatGPT, Claude, and Bard, built on LLMs, offer a myriad of possibilities right out of the box. There are two primary options to consider here: public tools and enterprise licenses. Public tools are the equivalent of public clouds, they’re readily accessible and easy to set up, but they offer less control over data privacy and customization. On the other hand, enterprise licenses function like private clouds, providing an extra layer of security and customization. These licenses often ensure that your company’s data is not used for additional model training, addressing privacy concerns similar to how a private cloud keeps your data in a segregated environment.
Tools based on LLMs are user-friendly and versatile; however, they may lack the ability to generate domain or company-specific content. Nonetheless, their low learning curve and quick time to value make them an appealing first step for many organizations.
Also, in the ever-evolving landscape of enterprise platforms, it’s crucial to stay nimble and open to change. Major tech players like Microsoft and Google will continually embed LLM tools into their platforms to provide more context for generic-use cases, such as summarization. To leverage these advancements, organizations must adopt an experimental mindset.
Context Matters: Leveraging Retrieval Augmented Generation
For businesses with unique terminologies or specific organizational context, RAG approaches can provide great value and differentiation. Unlike standard generative-model tools that deliver outputs based on the understanding of publicly available information, RAG models have the ability to add context from a company’s internal knowledge base. This means queries or content is created to align with your organization’s unique context and needs.
For example, if you’re in the healthcare sector, a RAG model could be configured to retrieve medical research, recent case studies, or proprietary data when generating content or responding to queries. As such, it can deliver highly context-sensitive and factual information that would be more difficult to achieve with a standard LLM.
Implementing a RAG model does require a commitment to more robust computational resources and a nimble development team. However, the moderate learning curve is often justified by the richness and specificity of the output, which can offer a time to value that is effective for businesses with specialized requirements.
Selective Customization: The Art of Fine-Tuning
Fine-tuning is a more advanced and higher-effort approach compared to a RAG model. While a RAG model can add recent organization-specific context, fine-tuning is about adapting the model’s core behavior itself. Notably, not all models are amenable to fine-tuning. For instance, Facebook’s open-source LLAMA 2 can be fine-tuned, but not all GPT models are built for this level of alteration.
With fine-tuning, you’re essentially rewiring the model to generate outputs that align more closely with a specialized dataset you provide. This is particularly beneficial for industries or use cases that have unique lexicons, strict regulations, or nuanced customer interactions. However, unlike RAG, where the focus is on retrieving and incorporating specialized information into the output, fine-tuning modifies how the model responds to all queries, not just specialized ones.
For example, a pharmaceutical company could fine-tune a model to analyze clinical-trial data. The model could be trained to understand the specific terminology and context of the company’s trials, enabling it to extract relevant insights more effectively.
It’s worth noting that fine-tuning a model to fit your specific needs requires a team with a good understanding of machine-learning techniques, and access to a relevant, sufficiently large dataset. The path to fine-tuning may be steep, with a high learning curve and a medium to long time to value, but the end result can offer a solution that is in deep alignment with your specific business needs.
A Bespoke Journey: Building from the Ground Up
When your business has highly unique needs, or the generative AI model serves as a core component of your product or strategy, then building a model from the ground up may be an appropriate path to consider. This approach requires a quantum leap in terms of effort and resources compared to the previous options.
While using an LLM might require minimal setup and configuration, fine-tuning or using a RAG involves a moderate level of expertise, and creating a model from scratch could demand significantly more resources. You’re not just training the model, you’re also developing the architecture, collecting and curating data, and undergoing rigorous testing and validation phases.
As an example, a medical research institute could build a model to predict the progression of a rare disease. Given the unique nature of the task, a custom-built model could potentially outperform existing models.
Creating a model from scratch requires a highly specialized team, significant computational power, and financial investment—as well as a long-term commitment. However, if a generative AI model is mission-critical to your business strategy—for example, if you’re developing a specialized medical-diagnosis tool—the high costs and steep learning curve can be justified.
Taking the Step Forward: There’s an Option for Everyone
The generative-AI landscape is vast, offering options that cater to varying levels of expertise, resource availability, and business needs. You might find value in the ease and immediate rewards of tools using LLMs or you might be considering the substantial commitment of building a custom model. Regardless, your solution will probably be a blend of these approaches and will certainly need to change over time as technologies evolve.
Our advice: start somewhere. Any step you take will bring you closer to unlocking new possibilities.
Both of us are deeply passionate about the transformative potential of generative AI, and we welcome the opportunity to engage with you further on this subject. Whether you’re just beginning your AI journey or have already made strides, we’d love to hear about your experiences and challenges. Reach out to explore the range of possibilities that generative AI can offer your organization.
Authors

Curt Basher
SVP, Information Systems
Curt Basher has been a key part of the Klick family over the past 12 years, with varied roles and experiences. With Klick fully embracing technology and specifically going all in on AI, Curt plays a central role in ensuring Klick is well ahead of the curve in AI use and innovation. That includes leading the Genome team through the evolution of the company’s award winning, home-built, and AI-rich platform. Genome is used by every Klickster daily and is truly a core part of Klick’s DNA.

Kamran Shah
EVP, Delivery & Solutions
Kamran has led multiple award-winning teams in engineering, product management, and marketing. Holding over 20 patents, his experience spans business-to-business and agency environments and he is passionate about transforming teams to successfully adopt technologies and business innovations.
Ready to Drive Life Sciences Forward?
Experience the transformative power of Klick Health, where deep industry expertise meets cutting-edge AI-driven wisdom.
As your trusted partners in life sciences commercialization, we combine a storied history in healthcare with the latest technologies to elevate every facet of your omnichannel strategy. From crafting engaging narratives to enabling data-driven decision-making, our integrated capabilities ensure you lead the way in transforming patient outcomes through digital health innovation.
Let’s create something transformative together.